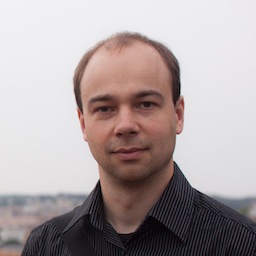
- Rafał Mantiuk
- Professor of Graphics and Displays
- Department of Computer Science and Technology
- The Computer Laboratory
- Rainbow Research Group
- University of Cambridge
- Office address
- University of Cambridge
- Computer Laboratory
- William Gates Building
- 15 JJ Thomson Avenue
- Cambridge CB3 0FD
- United Kingdom
- Room
- SS22
- Phone
- office: +44 1223 763831
- rafal [dot] mantiuk [at] cl [dot] cam [dot] ac [dot] uk
-
Important: if you have never sent or received e-mail from me, please include the text "n0t5pam" somewhere in the subject line, for example "[n0t5pam] Your subject". This is to avoid the SPAM filter.
If you are contacting me about internship, PhD studentship, or a PostDoc position, please check the "Jobs" section first.
Reserach interest
Applied visual perception; high dynamic range imaging; display algorithms; machine learning for image synthesis; tone-mapping; video coding for new display technologies; image and video quality metrics; visibility metrics; virtual reality and low-level perception; computational photography; computational displays; novel display technologies; colour; perception in computer graphics; novel image and video representations (beyond 2D); psychophysics; modeling visual perception with machine learning.
Biography
- Professor/Reader of Graphics and Displays, University of Cambridge, Computer Laboratory, UK (from 2018)
- Senior Lecturer, University of Cambridge, Computer Laboratory, UK (2015-2018)
- Lecturer/Senior Lecturer, Bangor University, School of Computer Science, UK (2009-2015)
- Postdoc Fellow, University of British Columbia, Canada (2008-2009)
- Postdoc, Max-Planck-Institut for Computer Science, Germany (2007-2008)
- Internship, Sharp Laboratories of America, Camas WA, USA (2006)
- PhD (summa cum laude, Computer Science), Max-Planck-Institut for Computer Science, Germany (2006)
- Msc (Computer Science), Technical University of Szczecin, Poland (2003)
Recent projects
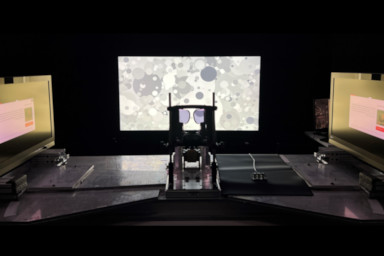
AR-DAVID is a video quality dataset that captures how distortions due to display technologies (e.g, waveguide non-uniformity) are going to be seen on an optical see-through AR display. We found a simple blending of the environment and display light cannot predict the visibility of distortions.
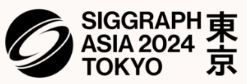
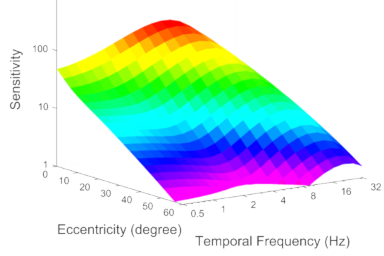
elaTCSF is a temporal contrast sensitivity model that can predict the visibility of a flicker, such as a flicker found in variable-refresh-rate OLED and LCD displays.
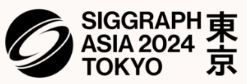
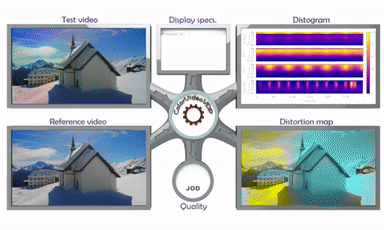
ColorVideoVDP is a differentiable image and video quality metric that models human color and spatiotemporal vision. It is targeted and calibrated to assess image distortions due to AR/VR display technologies and video streaming, and it can handle both SDR and HDR content.
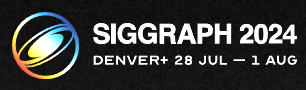
See more projects.
Recent papers
- Do computer vision foundation models learn the low-level characteristics of the human visual system?
Yancheng Cai, Fei Yin, Dounia Hammou, Rafał K. Mantiuk.
In: Proc. Computer Vision and Pattern Recognition (CVPR), 2025
[CVPR Highlight paper - top 14%]
(project page) (PDF) (code) - Training Neural Networks on RAW and HDR Images for Restoration Tasks
Andrew Yanzhe Ke, Lei Luo, Xiaoyu Xiang, Yuchen Fan, Rakesh Ranjan, Alexandre Chapiro, Rafał K. Mantiuk.
In: New Trends in Image Restoration and Enhancement (CVPR workshop), 2025
(arxiv) (PDF) - G-SemTMO: Tone Mapping with a Trainable Semantic Graph
Abhishek Goswami, Erwan Bernard, Aru Ranjan Singh, Wolf Hauser, Frederic Dufaux, and Rafal Mantiuk.
In: IEEE Access, 2024
(doi) (PDF) - Stereoscopic Depth Perception Through Foliage
Robert Kerschner, Rakesh John Amala Arokia Nathan, Rafał K. Mantiuk, and Oliver Bimber.
In: Scientific Reports, 14(23056), 2024
(doi) (project page) (PDF) - Modelling contrast matching across luminance levels
Maliha Ashraf and Rafał K. Mantiuk.
In: Color Imaging Conference (CIC32), 2024
(PDF)
See all papers.
Awards and grants
- HDR-VDP-2 paper received the Test-of-Time Award from SIGGRAPH (2023) for "a significant and lasting impact on computer graphics and interactive techniques over at least a decade"
- HDR-VDP-3 won the 1st place in the HDR Video Quality Measurement Grand Challenge (2023) in the full-reference category
- Color Imaging Conference 2022 (CIC30) Best Paper Award for the paper Suprathreshold contrast matching between different luminance levels
- London Imaging Meeting 2022 Best Paper Award for the paper A Comparative Study on the Loss Functions for Image Enhancement Networks
- ACM SIGGRAPH European Conference on Visual Media Production (CVMP 2022) Best Paper Award for the paper Distilling Style from Image Pairs for Global Forward and Inverse Tone Mapping
- Color Imaging Conference (CIC28) Best Paper Award for the paper Practical color contrast sensitivity functions for luminance levels up to 10000 cd/m2
- Electronic Imaging / Human Vision and Electronic Imaging 2020 Best Paper Award for the paper Predicting visible flicker in temporally changing images
- The IEEE Virtual Reality 2019 Best Journal Paper - for the paper Temporal Resolution Multiplexing: Exploiting the limitations of spatio-temporal vision for more efficient VR rendering
- Electronic Imaging / Human Vision and Electronic Imaging 2019 Best Paper Award for the paper A visual model for predicting chromatic banding artifacts
- ERC Consolidator Grant (2017) - EyeCode: Perceptual encoding of high fidelity light fields
- MSCA Innovative Training Network (2018) - RealVision: Hyper-realistic Visual Experience
- EPSRC research grant (2017) - A spatio-chromatic colour appearance model for retargeting high-dynamic-range image appearance across viewing conditions
- HPC Wales Research and Innovation grant (2013/14) - Video retargeting for delivery to mobile and future display technologies
- Royal Society Research Grant (2013) - Limiting factors of perceptual image fidelity
- EPSRC grant EP/I006575/1 (2011) - Quantifying image quality in computer graphics
- Heinz Billing Award 2006
My contribution to the organization of research networks and conferences can be found here.