Projects
AR-DAVID: Augmented Reality Display Artifact Video Dataset
Alexandre Chapiro(1), Dongyeon Kim(2), Yuta Asano(1), and Rafał K. Mantiuk(2).
(1)Reality Labs, Meta (2) University of Cambridge
Presented at SIGGRAPH Asia 2024, Technical Papers [Best Paper Award Honorable Mention]
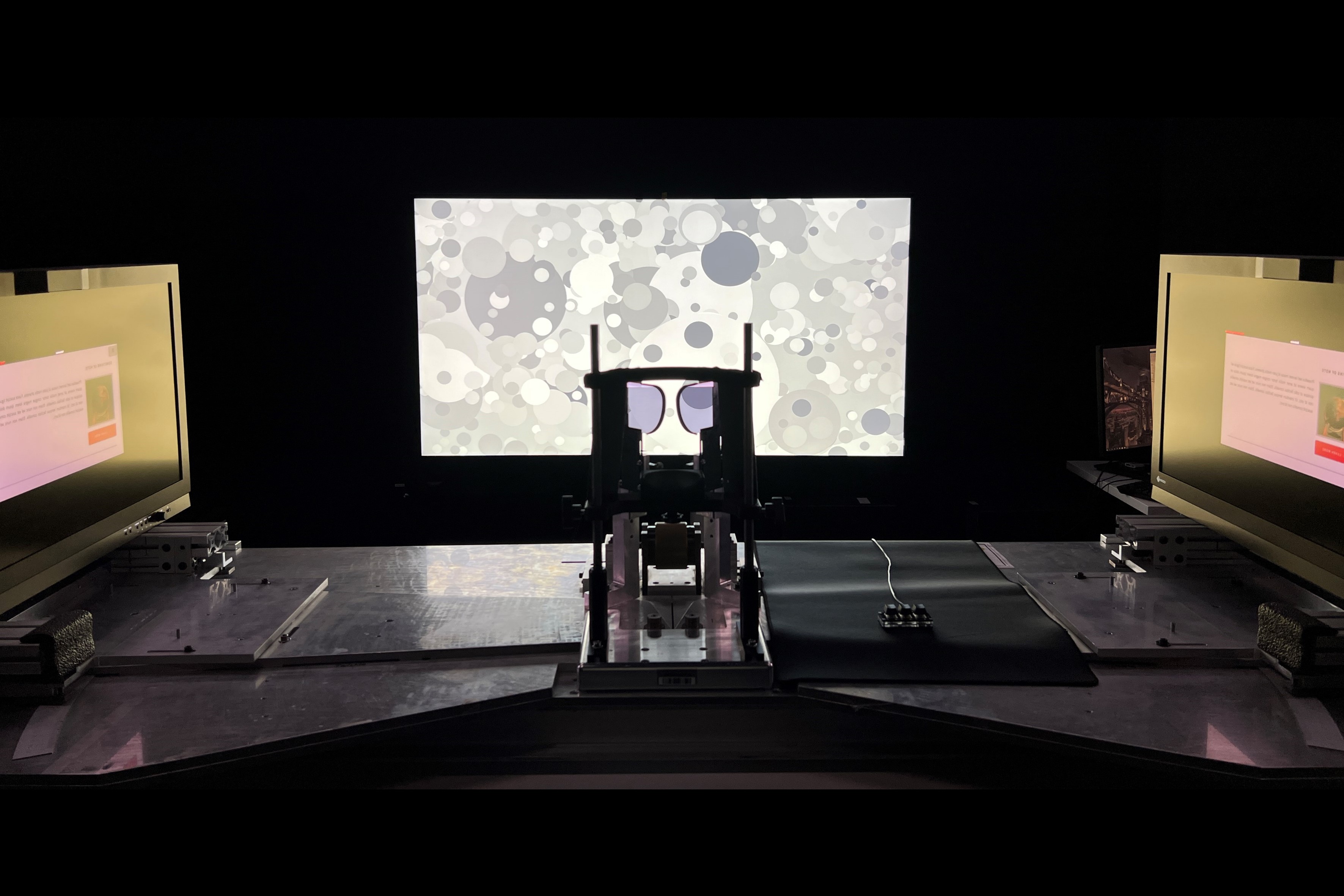
Abstract
The perception of visual content in optical-see-through augmented reality (AR) devices is affected by the light coming from the environment. This additional light interacts with the content in a non-trivial manner because of the illusion of transparency, different focal depths, and motion parallax. To investigate the impact of environment light on display artifact visibility (such as blur or color fringes), we created the first subjective quality dataset targeted toward augmented reality displays. Our study consisted of 6 scenes, each affected by one of 6 distortions at two strength levels, seen against one of 3 background patterns shown at 2 luminance levels: 432 conditions in total. Our dataset shows that environment light has a much smaller masking effect than expected. Further, we show that this effect cannot be explained by compositing of the AR-content with the background using optical blending models. As a consequence, we demonstrate that existing video quality metrics perform worse than expected when predicting the perceived magnitude of degradation in AR displays, motivating further research.
SIGGRAPH Asia 2024 Presentation
Materials
- Paper:
AR-DAVID: Augmented Reality Display Artifact Video Dataset.
Alexandre Chapiro, Dongyeon Kim, Yuta Asano, Rafał K. Mantiuk.
In SIGGRAPH 2024 Technical Papers, Article 186
[DOI] [paper PDF] - Code [Github (coming soon)]
- AR-DAVID dataset
Results
- Comparison of quality metrics [link]
This is a detailed report comparing the performance of 16 quality metrics, including additional metrics that could not be included in the main paper.
Related projects
- ColorVideoVDP - A visual difference predictor for image, video and display distortions
- FovVideoVDP - Foveated Video Visual Difference Predictor
- DPVM - Deep Photometric Visual Metric
- HDR-VDP - A Visual Difference Predictor for High Dynamic Range Images
- castleCSF - A Contrast Sensitivity Function of Color, Area, Spatio-Temporal frequency, Luminance and Eccentricity - models contrast sensitivity in ColorVideoVDP
- ASAP - Active Sampling for Pairwise Comparisons - used to efficiently collect AR-DAVID dataset
- pwcmp - Bayesian pairwise comparison scaling - used to scale AR-DAVID subjective responses