Data Science
Course arrangements
- Lecture notes
- Parts I & II as printed
- Part III as printed (distributed 29 Oct)
- Part IV as printed (distributed 12 Nov)
- Appendix: linear algebra
- Supervisions
Your college may have have arranged either three or four supervisions for this course. If three, the suggested breakdown is:
– Not for supervision: example sheet 0
– Supervision 1: example sheet 1
– Supervision 2: example sheet 2, and half of 3
– Supervision 3: half of example sheet 3, and example sheet 4
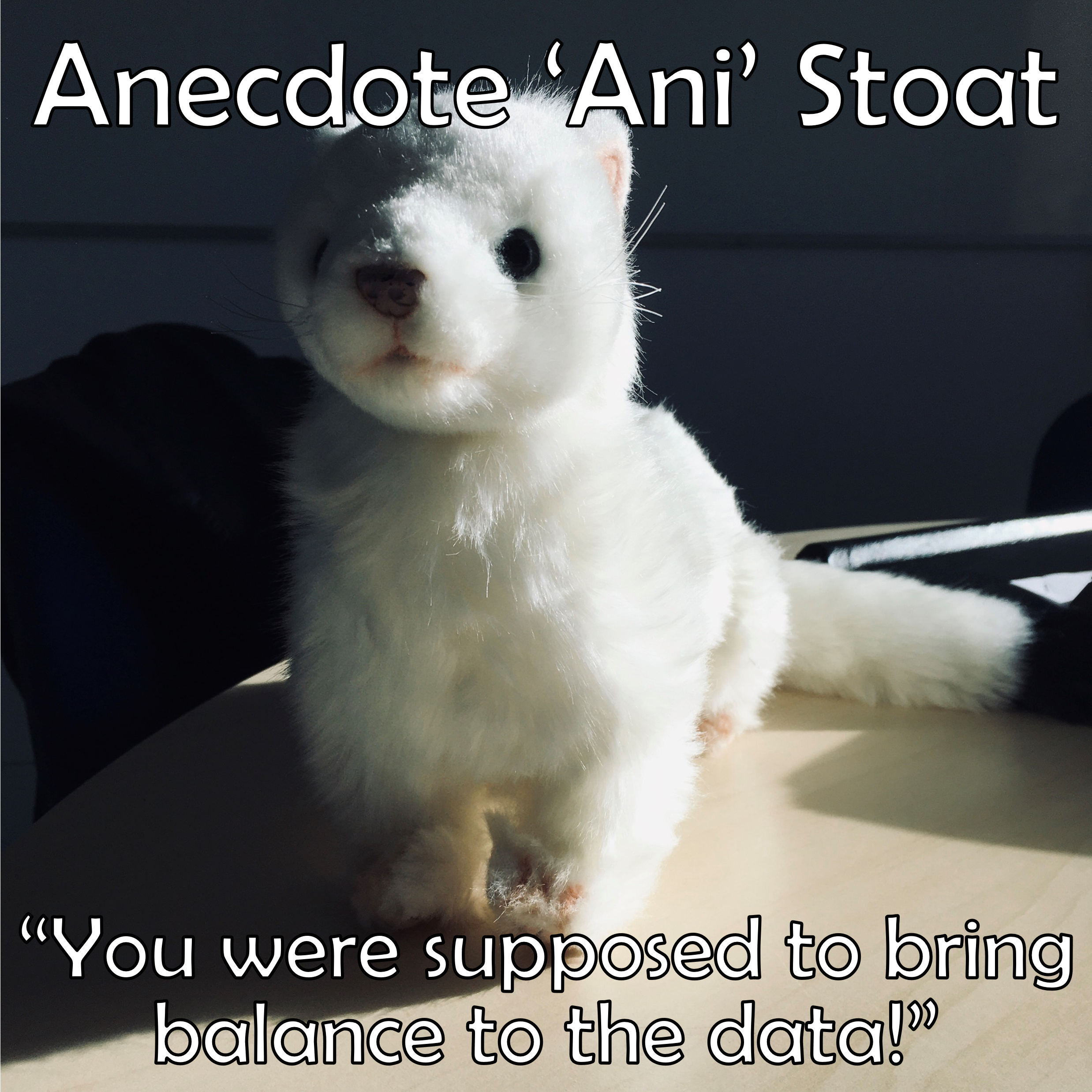
Videos and example sheets
The videos have exactly the same material as a normal lecture, but they are short because there's
no need for the lecturer to pause. You have a pause button — use it! You'll need to watch these
videos several times, with the printed notes beside you. When there are handwritten equations in the
videos, you should copy out the equations yourself to make certain you understand the method.
When the video presents code, you should download the code and try it yourself.
This will make the example sheets much easier.
—
The optional in-person sessions are digressions about off-syllabus topics and why it's all worth studying.
THE IN-PERSON SESSIONS ARE OPTIONAL. THEY ARE NOT EXAMINABLE MATERIAL.
Example sheet 0 and solutions (prerequisites) | |
Lecture 1 | |
8 Oct, 11am | Optional in-person: introduction [slides] |
Lecture 2 |
1.4 Numerical optimization (8:01)
|
Lecture 3 |
1.5 Likelihood notation (10:00)
1.6 Generative models (8:14)
fitting
1.7 Supervised learning (14:18)
fitting
|
Lecture 4 | Mock exam question 1 and walkthrough (23:36) |
15 Oct, 11am | Optional in-person: prediction and parameters [slides] |
|
|
Lecture 5 |
2.2 Feature design (19:39)
|
Lecture 6 | |
Lecture 7 |
2.6 Interpreting parameters (20:03)
|
Example sheet 1 | |
22 Oct, 11am | Optional in-person: climate dataset challenge [slides] |
Lecture 8 |
4.2 Bayes's rule calculations (15:37)
The printed notes have an error in Example 4.2.1. See extended notes for correction.
4.3 Deriving the likelihood (9:17)
|
Lecture 9 |
5.1 Monte Carlo integration (13:21)
7, 7.1 Bayesianism (16:54)
|
Lecture 10 |
7.2–3 Finding the posterior (24:45)
7.4 Bayesian readouts (6:28)
|
Mock exam question 2
and walkthrough (29:35)
|
|
29 Oct, 11am | Optional in-person: R2 and model choice [slides] |
Climate confidence challenge: find a 95% Bayesian confidence interval for the rate of temperature increase in the UK. Submit your answer on Moodle by 9am on Friday 5 November. | |
Lecture 11 |
6.1–6.2 Empirical cdf (15:10)
8. Frequentism (3:58)
|
Lecture 12 |
8.2 Hypothesis testing (23:37)
|
Lecture 13 |
Mock exam question 3
and walkthrough (18:20)
|
5 Nov | Optional in-person: the problem of induction [slides] |
Climate confidence challenge: find a 95% frequentist confidence interval for the rate of temperature increase in the UK. Submit your answer on Moodle by 9am on Friday 12 November. | |
Lecture 14 | |
Lecture 15 | |
Lecture 16 |
11.7 Drift analysis (11:23)
|
Mock exam question 4
and
walkthrough
(16:30)
Example sheet 4
|
|
12 Nov | Optional in-person: Bayesian versus frequentist? [slides] |