
of Computer Science
I am mainly interested right now in combining Machine Learning with Automated Theorem Proving.
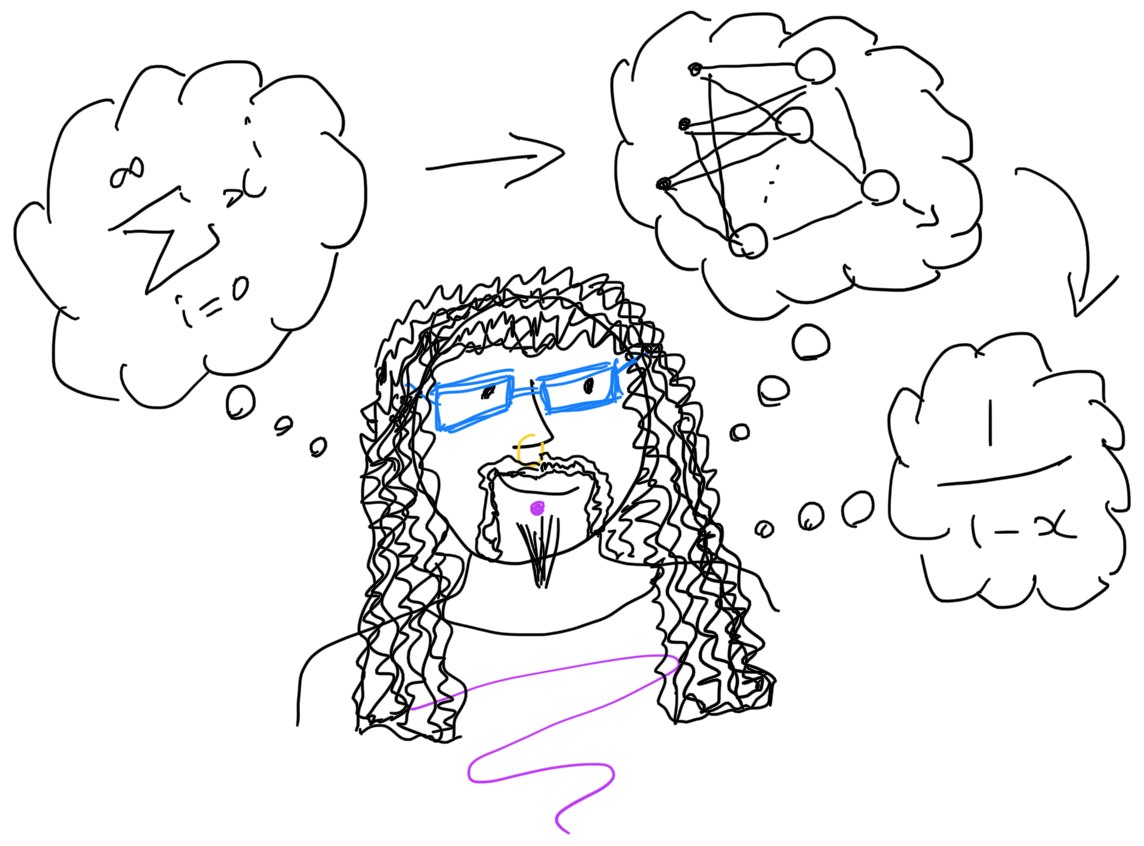
But I've researched in many different parts of machine learning, both pure and applied, over the years.
You can get a good idea of the kinds of thing I'm interested in from my 2021 half-day tutorial talk at CADE-28.
Research
“With 4 parameters I can fit an elephant. With 5 I can make him wiggle his trunk.” --- John Von Neumann, quoted by Freeman Dyson.
My research to date in machine learning (ML) and artificial intelligence (AI) is characterized by my career-long aim of maintaining breadth as well as depth in my approach to these subjects. Consequently I have a record of publication contributing to multiple areas across the theory of supervised learning, in particular in computational learning theory, and the application of multiple methods across multiple disciplines. For example:
-
My PhD and early postdoctoral research focused on understanding the ability of supervised learning algorithms to generalize. I have publications on classical Probably Approximately Correct (PAC) learning and on alternative approaches based in statistical physics. Further work followed from this addressing the quality of the theoretical results in practice. PAC bounds have tended to be too loose to be applicable. Some attempted to address this by, for example, turning to average-case analysis. I however became interested in the fact that they tended to employ the basic empirical estimate of error, while one might expect to obtain tighter results using the cross-validation estimate. This led to the first ever derivation of a PAC-like bound on the cross-validation estimate for a learning algorithm on a class of finite Vapnik-Chervonenkis (VC) dimension.
-
As a result of grant-funded research in collaboration with Glaxo-SmithKline, I was instrumental in the first published research applying support vector machines (SVMs) to a key part of the company’s approach to drug design. This led to multiple highly-cited publications.
-
I've been involved in the development of new algorithms in various areas of Bayesian inference.
-
More recent research in collaboration with the Department of Biochemistry addresses the localization of proteins within cells. We developed an algorithm capable of combining very high quality experimental data produced by the lab with much larger quantities of data of potentially highly variable quality obtained from the Gene Ontology. This was achieved in such a way that the more abundant, less reliable data did not overcome the smaller amount of good data, and in fact led to a significant increase in localization performance over the then prevailing state-of-the-art.
-
I also have a recent and ongoing interest in the potential of machine learning methods to improve the performance of automated theorem-provers (ATPs). In collaboration with Prof Lawrence Paulson and Dr James Bridge we demonstrated that machine learning can perform heuristic selection in the E theorem prover extremely effectively. I have published an extensive review of the current state of this area of research, specifically as it applies to SAT and QSAT solvers.
-
I have contributed to further research on the application of machine learning to other areas, such as sports science, medicine, signal processing and pervasive computing.
I am updating my site during November 2021, so you'll have to wait for more...
Current PhD Students
If you're interested in joining my group as a PhD student please read the FAQ.
-
Chaitanya Mangla: 1/10/2016 to present.
Subject: Machine learning for theorem-prover strategy construction.
-
Fredrik Romming: 1/10/2022 to present.
Subject: Machine learning for connection provers.
-
Francesco Ceccarelli: 1/10/2023 to present.
Subject: Graph Representation Learning for Biological Entities.
Previous PhD Students
-
Matthew Trotter, 1999 to 2006.
Thesis: Support Vector Machines for Drug Discovery.
Current position: Vice President, Predictive Sciences, Bristol-Myers Squibb
-
Andrew Naish-Guzman, 1/10/2003 to 22/04/2008.
Thesis: Sparse and Robust Kernel Methods.
Current Position: Quant Research Manager, G-Research
-
Ulrich Paquet, 1/10/2003 to 12/6/2007.
Thesis: Bayesian Inference for Latent Variable Models.
Current position: Research Scientist, DeepMind
-
Richard Russell, 1/10/2007 to 13/3/2012.
Thesis: Planning with preferences using maximum satisfiability.
Current Position: Software Developer, private hedge fund.
-
Nicholas Pilkington, 1/10/2009 to 3/12/2013.
Thesis: Hyperparameter Optimization for Multiple Kernels.
Current Position: CTO, DroneDeploy.
-
Ivo Timoteo, 1/10/2012 to 22/1/2019.
Thesis: Learning Dynamic Systems as Networks of Stochastic Differential Equations.
Current Position: Founder and CEO, Braid Technologies.
-
Agnieszka Slowik: 4/1/2019 to 2023.
Thesis: Out-of-distribution generalisation in machine learning.
Current Position: Microsoft Research Cambridge.