
AI and the City
Artificial Intelligence built into the fabric of the future city will dwarf the use-cases currently visible such as autonomous cars or home automation assistants, and the genie is already out of the bottle.
Ian Lewis, Director, Adaptive Cities Programme, Dept. of Computer Science & Technology, University of Cambridge, April 2019.
Artificial Intelligence has crept slowly into the light, particularly in a few highly visible use-cases. Tesla markets heavily the autonomous driving capabilities of their cars .. Amazon promotes its home voice-based query assistant Alexa. These are retail solutions, aimed at the consumer and consequently have narrow scope for the impact of their output. I.e. a badly driven Tesla might have serious consequences for a few people but the issue will be limited. An ignorant Alexa may buy you unwanted goods but your neighbor will be unaffected. Less visible are the rapid developments taking place in urban infrastructure and fewer commentators are considering the implications.
There's a lot of money in cities... a fairly basic transportation improvement can cost in the $billions while the GDP produced by a city can dwarf that of the rest of the country (the GDP-per-head of London is reported to be triple that of Wales). So the world's population is rapidly compressing into dense urban centers and the problems that can cause are leading to huge infrastructure spending. When an urban authority plans a $multi-billion infrastructure spend, a broadly accepted statement is "We have to spend some of that money being smart"™(my academic career is founded on that statement). In the developing world this program is evolving on the scale of entire new cities. The hard part is knowing what 'smart' looks like.
Here's an outline giving at least the direction of travel in global 'smart city' initiatives:
Sensor Networks. Urban sensor deployments are seeing orders-of-magnitude increases in the scale of deployment, from dozens to thousands. This is a quiet side-effect of the tech theme popularly known as Internet-of-Things. It is now normal at my University to engage with municipal authorities considering processing data from 10,000 cameras. This is sensor densification.
Real-time processing. There is an aspiration to process this urban sensor data in real-time. While this might sound obvious, the issues here are far more complex than deploying the sensors. Most if not all municipal authorities are limited to collecting the data with an intelligent human sifting through it afterwards, i.e. the data tends to be used for planning rather than real-time adaptation. For me the record was set reading an academic paper from New Delhi in 2018 which revealed half-way through that the air quality data was actually from 2010. This requirement becomes essential if the intent is to take timely action. If any of my colleagues from Wall St are reading this, when you get tired of processing FX Options prices in milliseconds you should come and work on real-time urban sensor data which is more fun and no-one else has a clue. This is the issue of timeliness.
Intelligent Sensors. Essentially the emergence of image-based sensors is transformational. The current go-to sensor for traffic information is a metal loop embedded in the road, the data from which (illustrated left below) says "blip" periodically. Researchers have tried to interpret the 'blip' without much progress. This can be contrasted with the detailed and granular information delivered by the image-based sensor illustrated on the right, essentially saying "I see 4 cars, 1 bus, 14 pedestrians". It can actually include flow rates for these and work is ongoing, for example, to analyze the 'pose' of the pedestrians. This is intelligent edge computing.
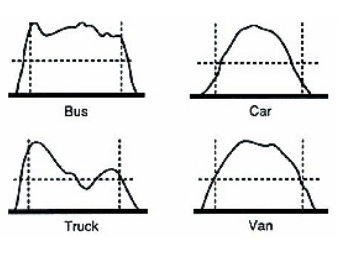

Prediction. The premise is simple: if you observed this real-time spatio-temporal data over an extended period (e.g. years), as a color-coded map showing traffic congestion for the entire city, continuously updating in real time, then you would get to know what normal looks like. A key point is this picture, shimmering like an oily pool, is varying in space and time i.e. what is normal for Greenwich Village is not necessarily normal for Staten Island and normal for 4am is not normal for 8am. but you'd work that out. Let's assume a computer could do the same thing and can spot something not normal in this traffic flow data. The natural expression would be 'traffic slower than usual' on the Jersey Turnpike, but note that the issue was recognized in both space and time. This alone might be useful, but if you think of macro area anomolus traffic flow as a pattern, the question is whether that pattern looks similar to one you've seen before, perhaps multiple times. In that case you have a reasonable chance of a data-driven prediction of what is likely to happen next. Performing these calculations in real-time permits the possibility of making adaptations to the city infrastructure (e.g. wide-area adjustment of traffic controls) before the otherwise inevitable more serious congestion occurs. This is machine learning.
Privacy. A significant challenge is that a variety of new sensors have the ability to intentionally or unintentionally track citizens through the city. Image-based sensors recognizing traffic flows can recognize the same vehicles elsewhere in the city. The same goes for pedestrians and cyclists. Information can be gleaned from combined sensor data sources (e.g. cameras and Bluetooth) that would not be apparent from each individual source. An ongoing area of research is to interpret human behavior from visual or audio sources, e.g. you can detect people fighting or arguing. If it turns out an arguing family is more likely to buy Prozac it is unlikely we should trust retailers not to exploit that information. Overall, it means that, like our Trust and Technology Initiative, you need a research program just to stay on top of the complex privacy issues.
Human-City Interaction. (CS pun intended) An important facet of all this technology is ultimately the citizens need to be able to interact with it in some meaningful way. This requirement is going to spectacularly increase if, as predicted, automated transport systems emerge where the urban infrastructure may have more influence over the individual journey than the passenger. Citizens concerned about their privacy may wish to query or question the data accumulated. In some circumstances the citizen may prefer to change behavior as a result of the real-time analysis (e.g. defer a journey). This is inevitably going to be the part of technology that lags behind the rest.
In summary, urban infrastructure has a lot of money behind it and is digitizing at a rapid pace. It presents a huge real-world use case for the emerging tech themes of the past decade, i.e. machine learning, big data, and internet of things. The consequences of this development will have an impact, probably on you personally.